When Evri launched their content discovery engine in public beta last week, it drew criticism from some top-tier bloggers for not having enough content. That’s probably true — the site is in beta and right now has a pretty narrow topic focus — but that is also likely missing the point about what Evri is trying to accomplish. I talked to Evri CEO Neil Roseman earlier this week to get an insider’s view on the Seattle company’s goals.
Evri is a semantic discovery engine. Roseman told me that it is their view that search is no longer sufficient for digging through the sheer amount of information out there. While Roseman stressed that Evri isn’t trying to compete with search — search does its job well, he said, and there is no real value for the end user in adding natural language processing to search — he doesn’t think that search does an adequate job in exposing relationships between the entities in the content it finds.
Rather than let users search for content, Evri uses natural language processing to try and make sense of content it finds and connect it, creating what the company calls the “data graph” of the web. The idea is something akin to the social graph that is exposed by sites like Facebook for our social connections, but applied to connections between entities mentioned in web content.
For example, Evri might look at an article about Blythe Danner, figure out that she is an actress, and then connect her to Gwyneth Paltrow, allowing users to figure out that Danner is Paltrow’s mother. Roseman tells me that right now Evri understands the how and why of many of the connections that it has uncovered, but they have not yet figured out a good way to expose that information to users on the UI end.
Of course, as with most current NLP search or discovery engines, the technology doesn’t work perfectly. The engine never seems to know as much about a topic as a well informed human, but it can point users toward connections that algorithmic search engines might have a hard time exposing. And that’s essentially the current goal. Roseman hopes that users will perform searches as normal on other search engines, then come to Evri to dig deeper and find related information about topics they’re interested in. He even thinks that Evri pages might find there way into traditional search engine results.
For now, Evri is building a destination site, with a focus on creating a simple and enjoyable user experience for exploring the connections its NLP engine finds. Ultimately, I get the impression that Evri hopes to be a back end technology where people build semantic search and discovery tools off the back of an API. Roseman tells me that the destination site they’re building will serve as a tech demo for developers — if users find value in what they’re creating, then developers will want in.
There are definitely some exciting future prospects in that. The Evri search page, which provides a peek into how the back end NLP analysis sees connections, also provides a look at the type of research applications that could be made on the back of an eventual Evri API.
A search for “[Organization/Name] > acquire > [Organization/Name]” for example, pulls up a list of companies that Evri knows have acquired or bought out other organizations.
You’ll notice a bunch of repeats in the results, but this sort of thing hints at the type of research applications possible with NLP discovery engines. Queries exposing connections could have powerful research applications — such as figuring out how many times Gwyneth Paltrow has worn Vera Wang to an awards ceremony, or how often a specific politician has visited a certain state, or how many times a band has played in a specific city, etc.
There is, however, a problem of scale. Right now Evri knows a good deal about politicians and celebrities, and Roseman tells me there are plans to expand the product’s field of knowledge to business, technology, health, and medicine. But adding that knowledge takes human power — creating and finding good seed lists, developing and curating ontologies, etc. The engine learns a lot on its own, but as I said earlier, I don’t think it will ever be as informed as a human expert on specific subjects. To expand Evri’s breadth of knowledge to cover all that Google can find might be impossible.
There is also a potential problem of physical scale. Unlike keyword indexes, natural language ontologies are generally larger than the documents they index. Because, for example, NLP engines have to try to figure out every pronoun and who it refers to in a document, NLP ontologies are necessarily large. As the number of entities grows, there is exists potential scaling issues.
Conclusion
Far from disappointing, I found Evri intriguing. While the destination interface is kind of clumsy, and I don’t know that Evri will ever fly as a consumer destination site, I am excited at the eventual prospect of applications being developed on the back of the company’s natural language processing technology and index of connections.
One smart thing that Evri is doing is offering widgets for bloggers that semantically analyze posts and point users to related content based on entities it finds in their writing. That will not only expose more people to Evri, but it will also bring more potential content into the engine.
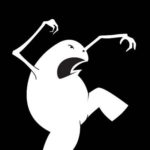
Before joining Jilt, Josh Catone was the Executive Director of Editorial Projects at Mashable, the Lead Writer at ReadWriteWeb, Lead Blogger at SitePoint, and the Community Evangelist at DandyID. On the side, Josh enjoys managing his blog The Fluffington Post.