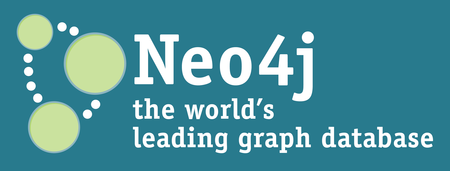
In a previous post, we briefly looked at the Neo4j database and created some simple Ruby API methods that leveraged our knowledge of the Cypher query language. We started to put together a simple CRM (Customer Relationship Management) application in an attempt to show how easily graph databases can navigate in a highly connected world. In this article, we’ll continue building on the CRM example by zooming in on the management structure of a fictional sales organization and see how easy it is to make complex queries of the database.
Key Takeaways
- Building a CRM with Neo4j and REST can be beneficial due to the graph database’s ability to handle complex relationships between data points, which is often required in customer relationship management.
- The process involves designing a database, expressing the data in a Ruby hash, and using the Ruby REST API to populate all this information into a graph database.
- Neo4j’s Cypher query language can be used to ask complex questions of the database, providing valuable insights into customer behavior and preferences.
- Despite the learning curve, Neo4j offers robust features, scalability, and a large community for support, making it a viable option for enhancing CRM capabilities.
Let’s Build the Database!
First, let’s build a simple database. Data for this database will be expressed within a Ruby hash structure. This hash structure could easily come from a JSON file, which, in turn, could come from a set of online web forms or an Excel spreadsheet.
This particular dataset will describe the structure of a sales organization and its relations to several client companies. In this structure:
- There is one territory manager who manages –
- Three account managers, each of which manages –
- One company account. Each company account has –
- One or more employees, most of which –
- Are managed by a manager.
- The database persists meetings between account managers and customers at their respective accounts.
The graph diagram below, which also appeared in part 1 of this article, describes the nodes and their relationships.
The Ruby hash structure that we’ll use to populate the database can be seen in the listing below. Note that, in the interest of simplicity, this is just a partial listing of the database. It contains sufficient information for you to understand where we’re heading. We include the @data
variable within the initialize
method of the RGraph
class, introduced in previous article.
@data = {
nodes: [
{
label: 'Person',
title: 'Territory Manager',
name: 'Linda Barnes'
},
{
label: 'Person',
title: 'Account Manager',
name: 'Jeff Dudley',
},
# ...
{
label: 'Company',
name: 'OurCompany, Inc.'
},
{
label: 'Company',
name: 'Acme, Inc.'
},
{
label: 'Company',
name: 'Wiley, Inc.'
},
{
label: 'Company',
name: 'Coyote, Ltd.'
},
],
relationships: [
{
type: 'MANAGES',
source: 'Linda Barnes',
destination: ['Jeff Dudley', 'Mike Wells', 'Vanessa Jones']
},
{
type: 'MANAGES',
source: 'Jesse Hoover',
destination: ['Ralph Green', 'Patricia McDonald']
},
# ...
{
type: 'WORKS_FOR',
destination: 'OurCompany, Inc.',
source: ['Linda Barnes', 'Jeff Dudley', 'Mike Wells', 'Vanessa Jones']
},
{
type: 'WORKS_FOR',
destination: 'Acme, Inc.',
source: ['Jesse Hoover', 'Ralph Green', 'Sheila Foxworthy', 'Janet Huxley-Smith',
'Tim Reynolds', 'Zachary Meyer', 'Milton Stacey', 'Steve Nauman', 'Patricia McDonald']
},
# ...
{
type: 'ACCOUNT_MANAGES',
source: 'Jeff Dudley',
destination: 'Acme, Inc.'
},
{
type: 'ACCOUNT_MANAGES',
source: 'Mike Wells',
destination: 'Wiley, Inc.'
},
{
type: 'ACCOUNT_MANAGES',
source: 'Vanessa Jones',
destination: 'Coyote, Ltd.'
},
{
type: 'HAS_MET_WITH',
source: 'Jeff Dudley',
destination: ['Tim Reynolds', 'Zachary Meyer', 'Janet Huxley-Smith', 'Patricia McDonald']
},
{
type: 'HAS_MET_WITH',
source: 'Mike Wells',
destination: ['Francine Gonzalez', 'Tsunomi Ito', 'Frank Cutler']
},
{
type: 'HAS_MET_WITH',
source: 'Vanessa Jones',
destination: 'Tracey Stankowski'
}
]
Using the RGraph
class, we’ll create some code to read in all the data and construct the graph database using the create_node
and create_directed_relationship
methods. The code for these methods was provided in the previous article.
Because there’s a lot of data to process, we’ll create plural forms of the above methods. More specifically, we’ll create two additional methods: create_nodes
and create_directed_relationships
that will be used to process each node and relationship expressed in the @data
hash. These plural forms will call their singular counterparts within looping structures.
def create_nodes
# Scan file, find each node and create it in Neo4j
@data.each do |key,value|
if key == :nodes
@data[key].each do |node| # Cycle through each node
next unless node.has_key?(:label) # Make sure this node has a label
# We have sufficient data to create a node
label = node[:label]
attr = Hash.new
node.each do |k,v| # Hunt for additional attributes
next if k == :label # Don't create an attribute for "label"
attr[k] = v
end
create_node(label,attr)
end
end
end
end
A main loop is set up in line 3 of the create_nodes
listing above. This loop simply looks for the node
array, which provides information for each node. Once this array is discovered, an inner loop begins on line 5. It filters out any nodes that do not have a label
attribute. The loop on line 10 is used to accumulate information for any node attributes. Finally, after gathering all necessary information, it calls the create_node
method, which, in turn, will push all this information into the Neo4j database.
A similar set of loops is used to create the relationships between the nodes. However, relationships are a bit more complicated because they need to unambiguously identify the nodes to which they’re attached.
The relationships are created in the create_directed_relationships
listing below:
def create_directed_relationships
# Scan file, look for relationships and their respective nodes
@data.each do |key,value|
if key == :relationships
@data[key].each do |relationship| # Cycle through each relationship
next unless relationship.has_key?(:type) &&
relationship.has_key?(:source) &&
relationship.has_key?(:destination)
rel_type = relationship[:type]
case rel_type
# Handle the different types of cases
when 'MANAGES', 'ACCOUNT_MANAGES', 'HAS_MET_WITH'
# in all cases, we have one :Person source and one or more destinations
from_node = {type: 'Person', name: relationship[:source]}
to_node = (rel_type == 'ACCOUNT_MANAGES') ? {type: 'Company'} : {type: 'Person'}
if relationship[:destination].class == Array
# multiple destinations
relationship[:destination].each do |dest|
to_node[:name] = dest
create_directed_relationship(from_node,to_node,rel_type)
end
else
to_node[:name] = relationship[:destination]
create_directed_relationship(from_node,to_node,rel_type)
end
when 'WORKS_FOR'
# one destination, one or more sources
to_node = {type: 'Company', name: relationship[:destination]}
from_node = {type: 'Person'}
rel_type = 'WORKS_FOR'
if relationship[:source].class == Array
# multiple sources
relationship[:source].each do |src|
from_node[:name] = src
create_directed_relationship(from_node,to_node,rel_type)
end
else
from_node[:name] = relationship[:source]
end
end
end
end
end
end
The array of relationships is located within the loop on line 3. Each relationship is then cycled, beginning with the loop on line 5. Note the filtering that takes place in lines 6 through 8, where we ensure that each relationship has, at a minimum, an identified type, a source node and a destination node.
The case
structure on line 10 differentiates between two types of relationships: those that have one source and multiple destinations, and those that have one destination with multiple sources. In cases where there are multiple sources or destinations, loops are used to cycle through each pair, and at the center of each loop is a call to the create_directed_relationship
method.
To finally populate the database with all this information, we instantiate RGraph
and create the nodes and relationships as shown below. Note that we must create the nodes before we create the relationships.
rGraph = RGraph.new
rGraph.create_nodes
rGraph.create_directed_relationships
The final result of all this work is shown in the Neo4j window below.
Before moving on to the final point, take stock of what we’ve done so far. We’ve designed a database and expressed the data in a Ruby hash. We then used the Ruby REST API to populate all this information into a graph database. Now, it’s time to ask the database some questions.
Query for Essential Business Information
Salespeople who work in B2B environments are very interested in understanding the hierarchical structure within their target accounts. The only way to get familiar with the hierarchy is to dig in and meet directly with the managers.
This particular database persists meetings between salespeople and individuals within their target accounts through the HAS_MET_WITH
relationship. The territory manager would therefore like to ask the following question: What managers have we not met within our target accounts? More specifically, the territory manager wants a list of managers that haven’t been contacted yet, the name of the company, and the responsible account manager.
For the sake of simplicity, we’ll abbreviate the Account Manager who works at OurCompany, Inc. as am
. Similarly, we’ll abbreviate each manager at the target account as tm
. Finally, we’ll abbreviate each targeted account company as tc
.
The Cypher query might look something like this:
MATCH (am:Person), (tm:Person), (tc:Company)
WHERE (am {title:"Account Manager"})-[:WORKS_FOR]->(:Company {name:"OurCompany, Inc."})
AND (am)-[:ACCOUNT_MANAGES]->(tc)
AND (tm)-[:WORKS_FOR]->(tc)
AND (tm)-[:MANAGES]->()
AND NOT (am)-[:HAS_MET_WITH]->(tm)
return am.name,tm.name,tc.name;
Okay, I admit I cheated here by throwing a WHERE
clause at you without warning. Similar to classic SQL, the WHERE
clause helps filter the results by adding a set of qualifications to the MATCH
.
The WHERE
clause breaks down as follows:
-
The account manager (
am
) is qualified by specifying that he/she is someone with the “Account Manager” title who works for OurCompany, Inc.
(am {title:"Account Manager"})-[:WORKS_FOR]->(:Company {name:"OurCompany, Inc."})
-
The target company (
tc
) is identified by virtue of the fact that it is account-managed by the account manager.
(am)-[:ACCOUNT_MANAGES]->(tc)
-
The target account manager is identified by the fact that he/she works for the targeted company (tc)
(tm)-[:WORKS_FOR]->(tc)
Also, the target account manager is further identified by the fact that he/she manages somebody.
(tm)-[:MANAGES]->()
The final parenthesis is empty in this case, because we don’t want to specify who is being managed; we just want to ensure that the targetedtm
is a manager. -
Finally, we filter on the fact that the account manager has not met with the manager at the target account. We use the keyword
NOT
to convey the negative relationship.
NOT (am)-[:HAS_MET_WITH]->(tm)
We write a quick method within Ruby that executes this query and returns the results as a JSON structure:
def find_managers_not_met
query = 'MATCH (am:Person), (tm:Person), (tc:Company)'
query += 'WHERE (am {title:"Account Manager"})-[:WORKS_FOR]->(:Company {name:"OurCompany, Inc."})'
query += 'AND (am)-[:ACCOUNT_MANAGES]->(tc)'
query += 'AND (tm)-[:WORKS_FOR]->(tc)'
query += 'AND (tm)-[:MANAGES]->()'
query += 'AND NOT (am)-[:HAS_MET_WITH]->(tm)'
query += 'return am.name,tm.name,tc.name;'
c = {
"query" => "#{query}",
"params" => {}
}
response = RestClient.post @url, c.to_json, :content_type => :json, :accept => :json
puts JSON.parse(response)
end
The final results appear as a Ruby hash as shown below:
{
"columns"=>["am.name", "tm.name", "tc.name"],
"data"=>[
["Jeff Dudley", "Jesse Hoover", "Acme, Inc."],
["Jeff Dudley", "Ralph Green", "Acme, Inc."],
["Mike Wells", "Mary Galloway", "Wiley, Inc."],
["Vanessa Jones", "George Quincy", "Coyote, Ltd."]
]
}
You can interpret the first data
array as, “Jeff Dudley has not yet met with Jesse Hoover, who is a manager at Acme, Inc.”
Summary
Graph databases are optimized to work with relationships between nodes. This objective immensely speeds up database queries when the critical information is relationship-based. Neo4j is one of the most mature and stable graph databases on the market. Although Neo4j provides libraries that port their database to every major language, including Ruby, it’s also possible – and fairly easy – to simply use the REST API instead of dealing with libraries. This necessitates becoming familiar with Neo4j’s Cypher query language. Fortunately, Cypher is fairly easy to learn, and it’s simple to place these queries directly in the REST API calls.
Frequently Asked Questions (FAQs) about CRM and Neo4j REST
What are the benefits of using Neo4j for CRM?
Neo4j is a graph database that provides a flexible and efficient way to store, process, and query data. It is particularly useful for CRM systems because it can handle complex relationships between data points, which is often required in customer relationship management. For example, it can easily track the interactions between a customer and various products, services, and employees. This can provide valuable insights into customer behavior and preferences, which can be used to improve customer service and marketing strategies.
Why are most CRM systems based on SQL rather than graph databases?
SQL databases have been around for a long time and are widely used in many industries, including CRM. They are well-understood, reliable, and supported by a large community of developers. However, they can be less efficient than graph databases like Neo4j when dealing with complex relationships between data points. This is because SQL databases store data in tables, which can require multiple queries and joins to retrieve related data. In contrast, graph databases store data as nodes and edges, which can be queried directly.
How does Neo4j compare to other graph databases?
Neo4j is one of the most popular graph databases, and it is known for its performance, scalability, and robust feature set. It supports ACID transactions, which ensures data consistency, and it has a powerful query language called Cypher that is designed specifically for graph data. It also has a large and active community, which can provide support and resources for developers.
Can I use Neo4j with my existing CRM system?
Yes, Neo4j can be integrated with existing CRM systems to enhance their capabilities. This can be done using Neo4j’s REST API, which allows you to interact with the database over HTTP. You can use this API to import data from your CRM system into Neo4j, and to query and update the data as needed.
What is the learning curve for Neo4j?
While Neo4j is a powerful tool, it does have a learning curve, especially if you are new to graph databases. However, there are many resources available to help you get started, including documentation, tutorials, and online courses. Additionally, the Neo4j community is very active and can provide support and advice.
How secure is Neo4j?
Neo4j has robust security features, including support for encrypted connections, role-based access control, and auditing capabilities. However, like any database, it is important to follow best practices for security, such as keeping the software up to date, using strong passwords, and limiting access to sensitive data.
How does Neo4j handle large amounts of data?
Neo4j is designed to handle large amounts of data efficiently. It uses a native graph storage format that allows it to store and query data directly, without the need for joins or other complex operations. This makes it highly scalable and capable of handling large datasets with ease.
Can I use Neo4j for real-time analytics?
Yes, Neo4j is well-suited for real-time analytics. Its graph structure allows it to query and analyze data quickly, even when dealing with complex relationships. This makes it a good choice for applications that require real-time insights, such as customer behavior analysis or fraud detection.
What kind of support is available for Neo4j?
Neo4j offers a range of support options, including documentation, tutorials, online courses, and a community forum. There are also commercial support options available for businesses that require additional assistance.
How can I get started with Neo4j?
The best way to get started with Neo4j is to download the software and try it out. There are many resources available to help you learn how to use it, including documentation, tutorials, and online courses. You can also join the Neo4j community to connect with other users and get advice and support.
Dan Schaefer is a web designer living in Ventura County, California. He’s been a part of several startups, doing everything from engineering to sales to marketing.